Episode 02: The Answer Is… Big Data
Join special guests Dr. David Classen, MD, MS, professor at the University of Utah School of Medicine, and Michael McShea, MS, MBA, group chief scientist at the Johns Hopkins Applied Physics Lab in the Health and Human Systems group of the National Health Mission Area. Discover existing solutions in applications of autonomous safety technologies and predictive analytics that could anticipate harm and intervene to prevent harm before it occurs.
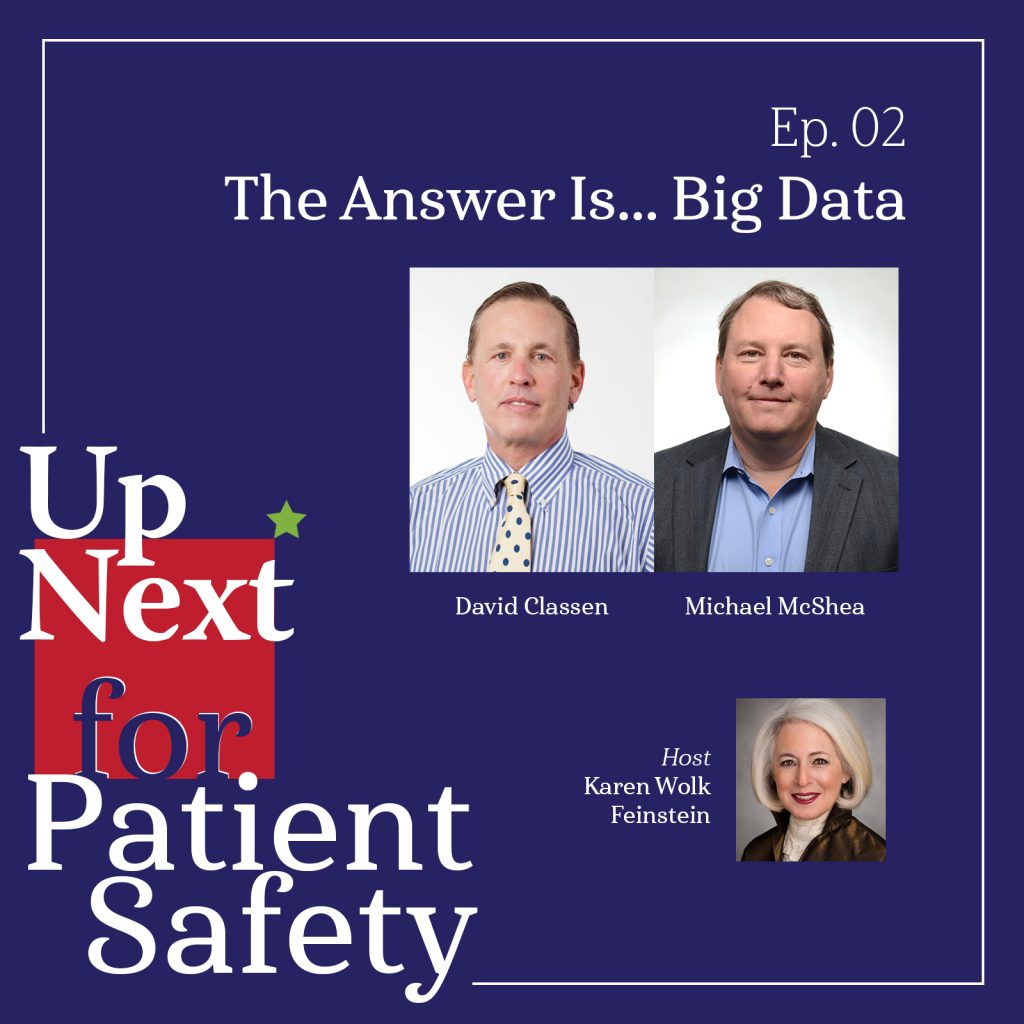
Listen to this episode on: Apple Podcasts | Spotify
Featured Speakers
- David Classen, MD, MS, Professor of Medicine (University of Utah); Chief Medical Informatics Officer (Pascal Metrics)
- Karen Wolk Feinstein, PhD, President & CEO, Jewish Healthcare Foundation & Pittsburgh Regional Health Initiative
- Michael McShea, MS, MBA, Group Chief Scientist of the Health and Humans Systems Group, Johns Hopkins University Applied Physics Lab
Referenced Resources (in order of appearance)
- Up Next for Patient Safety – Episode 01: Medical Error & the NTSB
- An Electronic Health Record-Based Real-Time Analytics Program For Patient Safety Surveillance and Improvement (2018, Health Affairs)
- Johns Hopkins University Applied Physics Lab – National Health Mission Area
- Centers for Medicare and Medicaid
- Fiscal Year (FY) 2022 Medicare Hospital Inpatient Prospective Payment System (IPPS) and Long-Term Care Hospital (LTCH) Rates Final Rule (CMS-1752-F) [Fact Sheet] (CMS, 2021)
- 10 years on from meaningful use, major progress despite the challenges (HealthcareIT News, 2019)
- Non-federal Acute Care Hospital Electronic Health Record Adoption (Office of the National Coordinator for Health Information Technology, 2017)
- Patient Safety Organization (PSO) Program (AHRQ)
- Patient Safety and Quality Improvement Act of 2005 (Patient Safety Act) (AHRQ)
- ‘Global Trigger Tool’ Shows That Adverse Events In Hospitals May Be Ten Times Greater Than Previously Measured (Health Affairs, 2011)
- Pascal Metrics Announces Groundbreaking Results – Virtual Patient Safety Solution Generally Available (Pascal Metrics, 2020)
- Project Firstline (CDC)
- University Affiliated Research Centers (UARCs)
- Johns Hopkins COVID-19 Dashboard
- APL’s Asymmetric Operations Sector: Driven by Envisioned Futures (Johns Hopkins University APL, 2021)
- Johns Hopkins Medicine – Armstrong Institute for Patient Safety and Quality
- Johns Hopkins Applied Physics Lab – MDIRA (Medical Device Interoperability Reference Architecture)
- U.S. Army Medical Research and Development Command (MRDC)
- National Emergency Tele-Critical Care Network (NETCCN)
- The Present and Future Champions of Patient Safety (ASA Monitor, 2021)
- Two Decades Since To Err Is Human: An Assessment of Progress and Emerging Priorities in Patient Safety (Health Affairs, 2018)
- Capacity Command Center Celebrates 5 Years of Improving Patient Safety, Access (Johns Hopkins Medicine, 2021)
- Up Next for Patient Safety – Episode 03: Paying for Safety
Episode Transcript
[00:00:00] Karen Wolk Feinstein: This is Karen Feinstein, I’m President and CEO of the Jewish Healthcare Foundation and our regional health improvement collaborative called the Pittsburgh Regional Health Initiative. So welcome today to Up Next for Patient Safety, where we attempt to untangle the web of causes for our high medical error rate and discuss promising solutions with experts.
As a reminder, if you haven’t listened yet, our first episode considers how can we adapt the National Transportation Safety Board model, the NTSB for patient safety and how that might dramatically reduce medical error. And we talked about how the autonomous solutions of the NTSB lessen the burden on frontline workers and management, think airbags, automatic safety brakes, autopilot, and so on.
And the NTSB solutions get widely adopted without shame, blame, regulations, penalties, or enforcement. So today I have a conversation with two experts in patient safety who are present two remarkable organizations that offer examples of how a National Patient Safety Board, our NPSB could improve safety.
If you’re wondering how… well, first by studying the major sources of harm from many different disciplinary angles. Second, analyzing large existing datasets to see what conditions precede these harms. And third, by proposing solutions before harm occurs, and by applying frontier technology and analytics. It’s no mystery this is what other complex high-risk industries do routinely. Joining me today I have Dr. David Classen and Michael McShea. Dr. Classen is an international superstar in health information technology. I’ve known David for 20 years and he’s taken me from worrying about the safety and errors of electronic health records to recognizing their amazing potential to improve patient safety.
David’s a Professor of Medicine at the University of Utah. He’s a Consultant in Infectious Diseases and he’s Chief Medical Information Officer at Pascal Metrics. David’s been involved in patient safety for three decades. Nationally, he chairs seemingly everything that’s safety and informatics related. He’s a prolific researcher and has authored peer-reviewed papers on medication safety, safety in pediatrics, health information safety, including the never-ending decision prompts that produce alert, fatigue, and even vendor safety.
I know you’ll hear more about his trigger tools. Our other superstar guest, Michael McShea, is a Group Chief Scientist at the Johns Hopkins Applied Physics Lab in the Health and Human Systems Group of the National Health Mission Area. It’s a mouthful, but it sounds very 21st century. He has degrees in electrical and systems engineering and a grounding in physics.
Michael knows a lot about digital health solutions, tele-health, informatics, health systems transformation and artificial intelligence. That’s why we consult him frequently. These two experts, excel at making complex concepts, methods, and technologies understandable. So here it goes. David, would you tell us something about Pascal Metrics, an organization that measures quote, “all harm for all patients all the time” end quote?
Okay. This is big data analytics on speed and timely. Given that the Centers for Medicare and Medicaid, the government agency responsible for more than 100 million people’s health care has just announced they are mandating the use of automated triggers by 2023. So tell us more about how Pascal Metrics prevents harm. And tell us about this big news and how your risk trigger monitoring is going to make healthcare safer.
[00:04:15] David Classen: Sure, thanks Karen and delighted to be here. The announcement by CMS, that they’re finally moving from patient safety measures that are based on retrospective administrative data to measures that are real-time and based on EHR data is a dramatic development in the industry. This has been a long time in the coming.
I’ve been working in this area for 20 years. And CMS knew that they had to replace their outdated administrative code-based measures. But I’m just delighted that they have finally moved forward and set a new direction for patient safety measurement in the United States. And it’s, I think taking advantage of a huge investment CMS has already made. CMS has invested more than $30 billion incentives for hospitals and clinics to put in electronic health records.
And it’s worked brilliantly. More than 95% of hospitals, and more than 90% of clinics now have electronic health records in place, which is a wonderful platform to start to move to the next generation of patient safety, which is to start leveraging all these electronic health record systems to measure safety in real-time when we can help patients while they’re still in the hospital – or in the clinic – and take the next step beyond that, which is to start to predict safety problems before they occur through the accumulation of this enormous amount of real-time safety data. So this transformation I’ve been working on for 10 years at Pascal Metrics, and Pascal Metrics is a federally certified patient safety organization and that law that enabled that passed back in 2005 and patient safety organizations have exploded.
Now, initially patient safety organizations mimic the safety world of hospitals, which was see something, say something i.e., safety was measured by voluntary reporting. And that occurred because we really didn’t have sophisticated electronic health records or advanced analytics that could start mining those databases for safety information.
So we really had to rely when people are voluntarily reporting. And unfortunately voluntary reporting, underestimates safety problems by about as much as 95%. So it was a natural opportunity with the broad adoption of electronic health records for any organization to start leveraging those records to measuring harm and that’s exactly what Pascal metric did. Through it’s patient safety organization structure, it was able to help hospitals join the patient safety organization and then measure harm electronically in a safe learning way where the hospitals were not penalized for discovering a lot more harm. And what Pascal has learned having done this in more than 200 hospitals in the United States and Australia, is that when they turn on electronic safety detection systems using data from the EHR, using artificial intelligence, machine learning and advanced analytic techniques. Hospitals can detect 10 to 20 times more harm than they have ever detected with all their existing systems. And this has been borne out in hospital, after hospital.
And so that allows hospitals to start tracking safety in a meaningful way that allows them to identify harm, not only at the hospital level, but down to the unit level. We never had enough data with voluntary reporting to find out that safety might differ in different units of the hospital. But Pascal has been able to show that and allow hospitals to identify that pressure ulcers might be a problem in this unit, but in the other unit, it might be DV deep venous thrombosis and another unit, it might be low blood glucose levels, in another unit it might be certain hospital acquired infections. So by increasing the amount of safety problems detected we’ve created, I think, or Pascal has created a meaningful measurement system that can be used over time for hospitals.
Not only to understand where the safety problems are, but also to measure their strategies, to reduce either overall harm or specific types of harm. And Pascal’s hospitals have demonstrated a reduction of all-cause harm as much as 64% and a reduction to specific types of harm. Let’s say oversedation by as much as 80%.
So, as the old line goes, “you can’t manage what you can’t measure.” This is absolutely accurate, right? Once you put a better measurement system in for safety, hospitals can make meaningful reductions in safety. And that’s important because we’ve been working on the safety issue for 20 years and all that we’ve had are narrow areas of improvement.
Reducing all-cause harm has not really occurred yet. So, I think IT offers an incredibly promising approach to do this. But when you start to build this system, it’s not just the software, but it’s also how you monitor and measure. And so, coming up with effective means that allow hospitals to monitor and measure all patients rather than just a fraction of them, which is what we did before with our voluntary reporting, has been a challenge, but we’ve been working on it for 10 years and have created a fairly sophisticated review system that allows us to review every patient, every day.
And indeed you can extend that even further. Pascal has developed a 24/7 monitoring service that allows hospitals to have all their patients monitored 24/7 and takes the burden off hospitals to have to do all the review themselves. Someone off-site can do that. And we’ve seen this in a lot of other industries, these remote monitoring centers, and that is now taking off among Pascal clients, which is remote monitoring that can not only measure consistently and accurately and reliably, but also in real-time, can show hospitals patients that need to be intervened on. And then the next step in this journey that Pascal has taken is if you collect all this information and unify a large database, you can develop predictive analytic programs that can predict the harm before it’s occurred. And Pascal has demonstrated the ability to predict harm up to three days before it occurs in in hospitalized patients. So that’s sort of a quick summary, Karen, of what Pascal has been doing in this space and I’ll turn it back over to you.
[00:10:54] Karen Wolk Feinstein: That’s impressive, David. I’m sure at first, how systems don’t always want to know and are somewhat shocked at their true error rate, but when you wrap it all together in a package for improvement, basically autonomous improvements, it’s more palatable, but we may get back to that later. It’s… I’m sure maybe one of the sticking points on why progress has been slow.
Let me turn now to Michael. Would you tell us something about Johns Hopkins Applied Physics Lab? As a multidisciplinary research and development organization, APL somewhat resembles our vision of an NPSB, but you’re enormous, with over 7,200 employees, though, many are devoted to safety in defense and space travel.
Tell us about your national health mission at APL. What do you do in health care and how do you engage many disciplines such as, environmental science, infectious disease, computational physics engineering, molecular biology, genetics on your innovation teams? If you get a chance, you might mention Project Firstline and your work to reduce dangerous airborne disease.
[00:12:06] Michael McShea: You’ve summed up APL very well but let me just say a couple things, because I think most everybody has heard of Johns Hopkins, but many people have never heard of APL. And so, what we are is called a university affiliated research center, which acts as a trusted arm of the government. So, Johns Hopkins university and Johns Hopkins Medicine are like sister organizations to us.
And we basically serve to maintain capabilities around national defense, but with all those skills we put them to use for other national good as well. And national health mission area is one of those areas. We do collaborate very closely with Johns Hopkins Medicine. Everyone just about everybody has seen the COVID dashboard, I think over the last year. That was a collaboration between APL and Johns Hopkins university in medicine. Firstline is another good example of that and our in-health precision medicine platform is another area where APL has worked together with Johns Hopkins Medicine. But just to briefly touch on APL and what we’re all about, it’s all about taking new technologies and capabilities all the way from the pure sciences side of things – and you mentioned a lot of the disciplines associated with that – all the way through to actually creating operational capabilities around those technologies. And that’s really the applied part of Applied Physics Lab. We happen to sit in a sector of national health that is in APL called the Asymmetric Operations Area and Sector. And that basically is all about asymmetric – when you think about it – is applying a lever that has a large outcome, a large effect, and so that’s what I’m excited about in national health is how can we use technology as a lever of change and especially in patients. And I think that there’s lots of different disciplines that can be brought to bear to those problems as you mentioned.
And so Firstline is a great example of this and I appreciate you bringing that up. I think many of your listeners may already know what Firstline is, it’s actually a CDC program around improving – or I should say – preventing infections in hospitals and so forth. And so, what Project Firstline is that we’re involved with, it’s a collaboration with the Armstrong Institute, which is a Johns Hopkins Medicine Institute around patient safety that many may have heard of as well. And we’re basically really analyzing in depth from many different angles, the operating room environment, for starters and the environmental airflow and aerosol effects of how infections spread in those environments.
It’s a good example really of how you take lots of, of pure science type capabilities, whether it’s the physics of how aerosol spreads in the air, the microbiology of what’s in the air, even down to DNA sequencing, and put that together with some advanced modeling and data science capabilities, bring in sensors to actually model the entire environment and then put that to good use. Measuring things like how the infection spreads from patient-to-patient. Looking at aerosol generating procedures that are there – of which there are many in the operating room – and how to protect providers in that situation and even looking at the actual procedures that are used to prevent infection control and actually physically measuring the effectiveness of those procedures.
And as you pointed out, I think in the human factor side of things, it’s a whole dimension of this, it’s not just about physics and chemistry and biology. It’s about how humans interact with the technologies and the procedures to begin with and so human factors and cognitive sciences are very much a part of what we do.
[00:15:31] Karen Wolk Feinstein: I can see – thank you, Michael – I can see how Project Firstline’s work not only addresses the movement of a virus like COVID during a pandemic, but I mean, it could be deployed to protect both patients and workers from infectious disease at all times. So, it’s kind of both a medical and a public health innovation.
This is exciting. It suggests that NPSB solutions have multiple applications. Let’s think a little bit now about autonomous solutions that both of you have somewhat mentioned, and what we hope will be an outcome of an NPSB. So, David, could you give us a quick description and a definition of these terms we throw around artificial intelligence, machine learning, autonomous solutions.? And also tell us, how do you use data from EHR and other existing sources to take the burden off frontline workers and even David, to empower patients?
[00:16:28] David Classen: Just basically a summary of artificial intelligence and machine learning, using a concrete example. So we have collected at Pascal, hundreds of thousands of examples of patient harm and documented them all relentlessly from EHR data. What we needed to do was find a way to analyze that data so that we could predict harm before it occurs. And so we use techniques of machine learning to help us analyze that data.
We use techniques of artificial intelligence to develop predictive models that could build on the analysis of that data. And then ultimately to create an application, if you will, an artificial intelligence application, that would basically, predict in every patient in the hospital, what their risk of harm was as frequently as every hour. And that led to the development of a global safety risk predictor that when we studied it was able to predict harm up to three days before it occurred. And so we used a whole variety of analytic techniques in artificial intelligence and machine learning to build those models.
And I won’t go through them in great detail, but these techniques, on one level on our extension of statistical techniques we’ve been using for years, they’re just more sophisticated. So rather than doing logistic regression and linear regression, you can go farther with much more sophisticated techniques and machine learning to analyze that same data. With the intent of developing complex models that can be used to implement something as simple as predicting safety problems ahead of time. So I would say, as many people think AI is something brand new, no, it’s an extension of sophisticated data analytic techniques we’ve been using for a long time. That said, it clearly is more effective in developing predictive algorithms than are old analytic techniques of simple linear and logistical regression.
[00:18:33] Karen Wolk Feinstein: Thank you, David. Okay, Michael, you knew I was going to ask you about MDIRA. M-D-I-R-A, and for our listeners, I am not obsessed with a woman, but with a life-saving pod. So Michael, tell us, how do you apply artificial intelligence, machine learning, and advanced diagnostics to keep wounded warriors alive on the battlefield. And could we ever use MDIRA at our bedside?
[00:18:59] Michael McShea: I’m happy to talk about it. It’s a, and yes, MDIRA kind of sounds like a Greek goddess, but it actually stands for Medical Device Interoperability Reference Architecture, which is a mouthful. But think of it this way, it’s all about building autonomous medical systems, which of course has a pretty strong AI component to it. And you’re right, the end vision is actually literally robotic field care in the battlefield for extended periods of time with no humans available. But there’s actually a lot more relevance to it and nuts and bolts to it that’s really germane to this discussion, because it all starts with data, right? And the data and the interoperability of that data, and the ability to harness that data through algorithms to develop AI, to drive these autonomous systems to begin with, right.
And so it really does go all the way down to the bits and bytes of data for medical devices all the way up through the AI and the artificial intelligence built into robots, quite literally. But when we… long before we think about robots, what we’re really thinking about is how do we make a medic in the field effectively have superpowers, right? That’s what it’s really about. It’s how do you help them do procedures that they’re not really trained to do? How do you help them know what procedures need to be done to begin with and what kind of medical device capabilities, automation do you provide that helps them take care of multiple wounded at once? Without having to attend to all the decisions that need to be made around ventilators, infusion pumps, and all those things over time.
So that’s what it’s all about. It’s all about the superpowers that AI can provide that medic. And I think that that kind of brings it home a little bit. I think we’re all kind of familiar with the term autonomous, or automatic or that sort of thing. We all have heard about autonomous driving and cars and things like that, right, and autopilot on an airplane. Well, take that concept and bring it into the medical context of how a clinician delivers care to a patient. And that’s really what MDIRA is trying to enable. I’ll say one more note about this, and this is by the way, a program, it’s an army program funded out of the Medical Research and Development Command, the MRDC. Another program from that outfit is called NETCCN, the National Emergency Tele-Critical Care Network. And that one’s actually a lot closer to home and it’s lot closer to now. Anyone familiar with tele-critical care knows that it’s all about how you, basically, operate an ICU remotely and how you provide remote control, how you provide clinical decisions support. So NETCCN is really all about responding to something like COVID with technology and interoperability and MDIRA is a fundamental part of that.
[00:21:35] Karen Wolk Feinstein: Thank you, I hope people are as convinced as I am that there’s something magical here in MDIRA and the surveillance, the diagnostics, prediction, corrective actions. All amazing and autonomous. So many other complex high-risk industries have embraced safety technology built on some of these principles.
I keep wondering, why did doctors fear artificial intelligence and big data? Where does this fear come from? How could we respond and gain acceptance? So David, you know doctors hate their electronic health records and you were an early warning system for what could go wrong. However, as I said before, you now see enormous potential. The data are here, the EHRs will get better and you can help us use what we have.
Could you cite some examples? I’m thinking often of the anesthesiologist. They’re the one specialty I know that have really embraced artificial intelligence, machine learning, human factors engineering to make care enormously safer. How do we get others to buy in? And why do the anesthesiologist jump in and have stayed in?
[00:22:49] David Classen: Yeah, the anesthesiologist have probably been the leaders in patient safety for most of this focus over the last 50 years. They really did adopt the principles of safety but more than 50 years ago, more than any other specialty. So they’ve been primed with a special interest in safety, over most of the other medical specialties, Karen.
So when all these new techniques arrive, they were already, I think, well-accepting and well-versed in how they might play a role in improving the safety of their use. And if you look at complications from anesthesia, I mean, they’re on a level with complications from air flight, right? And yet, in other areas of medicine, we have much higher rates of harm.
So, anesthesia, I think, was the national and natural first step in this, but it is possible to engage other physicians and I’m going to hark back to a project we did back in the nineties at Intermountain Healthcare, where we used machine learning and artificial intelligence, pulling data out of our own homegrown EHR to make a doctor’s complex task, much simpler and data driven. And that was the complex task of prescribing antibiotics and antifungals and antiviral medications in patients who might have an infection. And traditionally, it was all paper-based, right. Doctors ran around with a little pocket thing called the Sanford guide with teeny small print you couldn’t read and thousands of pages, and would run through that to try to decide what to prescribe. We said, “gee, why don’t we just automate this?” Why don’t we create a system that pulls all this data from the EHR and can help the doctors, in real-time, with this patient at this time prescribe the right antibiotic or antifungal or antiviral.
And initially we thought doctors would be really resistant to this. But guess what, we demonstrated in a clinical trial that it actually improved the quality of care, published in the New England Journal, and after that, it had great credibility with our doctors. And once they started to grab on to it, you couldn’t take it away from them because what it did is, it took a complex task that might take 20 minutes to hand gather all the manual information… and it took only a couple minutes when we pulled it all together electronically and it provided real useful guidance to physicians. So I think that’s an example from 20, 30 years ago, almost the problem in healthcare is we have not replicated that with all our EHRs. And why haven’t we done that? Because the goal of the EHRs from the point of view of basically our healthcare financing system is to track stuff to justify payment, rather than to provide tools for doctors to improve. And I get that, because our EHRs, have been turned into giant billing machines. But we’re making a transition now, as we get smarter and more effective about providing value through the EHR, that’s usable and solves problems, and makes doctor’s tasks more efficient and more effective.
So I think we’re at that golden era where we’ve put the platform in place, we’ve done all this stuff we had to for billing, and now we’re moving on and optimizing into these areas. And so I’m really optimistic about where this goes in the future.
[00:26:03] Karen Wolk Feinstein: Well, not only have you convinced me of the value, but you’ve given me hope so… so Michael, let me ask you, how does systems engineers deal with technology hesitancy and from your experience, where are some dramatic opportunities for safety breakthroughs?
[00:26:21] Michael McShea: Funny you should ask about technology hesitancy when there is vaccine hesitancy going on, right. I think the answer in there, in part, lies in rarely marrying the cognitive sciences together with the engineering sciences and at APL, we do a lot of work in human-machine teaming and it’s a little bit more interesting than it even sounds in that you’re really trying to understand, not just how the human’s mind works relative to the technology, but the technology under it needs to understand how the human mind works. And it actually is really interesting, but when you pair, you put machines into game situations where they’re paired with a human, they do more poorly than when they’re paired with other machines, because they don’t understand well enough yet how the human mind works.
And so, to some degree, it is a human factors engineering problem to solve, but there is another really key dimension to it and that is the human dimension. And that’s where the cognitive science comes in. The clinicians need to trust the technology. And, I think as you all well know, I mean… doctors are scientists, right?
They want to understand not just that it works, but how it works, and they want to interact with it and have the right information to actually make the decisions. And not just take an alert from a black box that says you need to do something. And so it really is a science in itself to really understand what is needed by the clinician to trust the technology.
And to know what technology that maybe isn’t serving them well and that sort of thing. But I think that the other reason there’s a trust issue is this notion that somehow AI is going to replace doctors. And I think the really interesting thing that we find a lot of the time is that the AI may perform better than the clinician in some cases, but the AI together with the clinician performs better than either the clinician or the AI.
And so we really need to start thinking about this as an ongoing assistant to the clinician. And we’ll get more trust that way and we’ll get more adoption that way, but the trust issues are real. And we’re not going to get the kind of breakthroughs we think we can without passing that hurdle.
And now on the safety breakthroughs where I think the dramatic opportunities are, I think that David’s already said it to a very large degree. We’ve spent the last 10 years digitizing all of our healthcare data and that is creating a huge opportunity to put that data to work for patient safety.
And I think David’s already done a really good job of describing that. Taking it a step further, there’s a couple of really interesting things to think about, and that is, what about all these mobile apps and wearable devices that can collect our medical information when we’re not sitting in front of a doctor, and how do we do something with that to make it – not just safe when you’re doing a procedure in an operating room or you’re in the hospital for something – but to make it safe when a doctor is managing your condition with a lot more data to work with. So those are both really dramatic opportunities to change the game in patient safety.
[00:29:13] Karen Wolk Feinstein: Thank you. And I did definitely mention technology hesitancy in the same way that I’ve been talking about vaccine hesitancy. If you don’t understand the full picture and you’re not well-informed, as David said, you just look at more errors surfacing instead of actually relieving your frontline staff, but not only that, taking care of your patients in a way that is dramatically better and safer.
So let me ask both of you because you’ve thought about patient safety for decades. If we ever had a National Patient Safety Board, which I hope we will, what do you see as some of the most promising areas of research? Where might you begin to study? What are the harms that create some of the major setbacks for patients as well as death and disability? So, David or Michael, whoever wants to go first.
[00:30:10] Michael McShea: In terms of research that could be done, I think there are huge gains to be made in trying to figure out where and how AI technology can scale across the industry and really understanding the obstacles to that happening. And I think without an NPSB, it’s really just unlikely to happen.
And on that note, I would say as well that we have all this data and we have over time, we’re going to have more and more, AI or machine learning, deep learning algorithms, neural network algorithms that are actually producing all kinds of additional information. And so what does that mean? That means that not only do we need really good surveillance technology… it’s too much information for humans to actually process so we need surveillance technology, but we also need almost a whole ‘nother level of surveillance involving the human part of this. And that’s where I think command centers come into play.
There are lots of interesting things happening in this space already as you all well know, but this is not a new concept. Other industries have operation centers and command and control centers for a whole wide variety of things. Think of cyber operations when you have potentially millions and millions of attacks happening at one time. And you think of a hospital situation where there’s all kinds of things happening at one time that can be monitored, but how do you actually leverage the kinds of technologies used in other industries to really have the appropriate command and control of what’s going on in all those environments?
I think that would be really interesting. And I think some research about how standing up those capabilities changes the game. Not just the introduction of AI at the care provider level, but harnessing that at a whole health system level. I think that kind of research would be really helpful in pointing the way of where the investments are needed.
[00:31:54] Karen Wolk Feinstein: Thank you, Michael, I’m also underlining the fact that we have quite a few command and control centers now with sensors and monitors and access to all the data and electronic health record. We’re just focusing them on payment and financing. As David said, that’s how we use our electronic health records, but they could be purposed for safety, so thank you. David, do you want to add to that?
[00:32:21] David Classen: Yeah, I completely agree with Michael and with you that most of our technology initially in healthcare gets adopted related to billing and payment. And nowhere, is that more clear than in the current monitoring centers that are focused on logistics and everything else, but because we’ve automated all of, most of the electronic health record in the inpatient/outpatient, we now could turn those monitoring centers into real-time safety monitoring centers not only for the inpatient, but also the outpatient.
And why do I mention the outpatient? Well, the inpatient is much more closely overseen for patients, but remember patients spend most of their life in the ambulatory setting. And so that if you want to know where the greatest opportunity to improve safety is to use these remote monitoring centers to help patients at risk in the ambulatory arena and all the data from EHR, as well as the sensors Michael was talking about, gives us an incredibly rich data source to start to begin to support patients outside the hospital. So I would say one of the greatest opportunities is to apply this idea of remote monitoring and support patients in the ambulatory settings of care, especially for high risk patients.
[00:33:35] Karen Wolk Feinstein: Well, I can’t thank you both enough. You’re always available to us and you’ve provided us with so much guidance as we’ve gone along in our journey. And I think you offer a lot to this country in some of your breakthroughs, I will just wind up by saying to any of our listeners. If you want to get engaged with promoting a more permanent solution, like the NPSB, the National Patient Safety Board, we do have a national coalition made up of representatives of all the stakeholders including, providers, insurers, consumer action groups, and more. We’d love to have you join us. If you want more information on either how to join the coalition or more background information on patient safety, please go to our website, npsb.org. And also listen to the other podcast episodes.
We cover issues such as, as I mentioned, the National Transportation Safety Board… what is it and why is it so powerful? We’re going to be looking at our financial and payment systems and how little incentive our current systems offer for hospitals and health systems to take on the expense of additional safety interventions… but that may be changing. So, we will have a podcast on what value-based payment might mean for a greater demand for safety interventions. And we’re also going to have a podcast on human factors engineering (link to subpage coming soon). Every other industry applies human factors, engineering to safety and we’ve been rather sluggish in healthcare. Very few of our health systems have a human factors engineer anywhere up near the executive suite, but that may be changing also.
So thank you all for listening. Thank you so much, David and Michael for your ongoing wisdom. And hopefully we’re going to start to make some important change. Thank you.
—
Subscribe on your favorite podcast app: Apple Podcasts | Google Podcasts | Spotify | Pocket Casts